Aug 02, 2024 | AI
How Harmful Are Errors in AI Research Results?
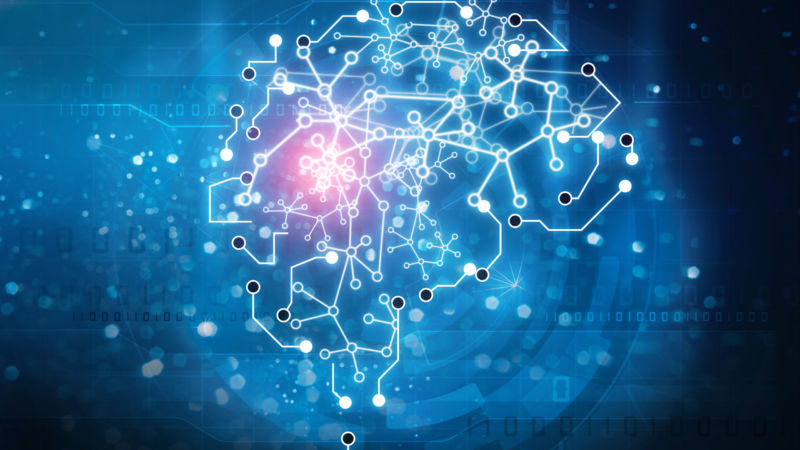
A guest post from Mike Dahn, head of Westlaw Product Management, Thomson Reuters
AI and large language models have proven to be powerful tools for legal professionals. Our customers are seeing the gains in efficiency and tell us it’s greatly beneficial. However, there has been a lot of discussion lately of errors and hallucinations, but what hasn’t been discussed is the extent of harm that comes from errors or the benefits of answers with an error.
First, let’s settle on terminology. We should use terms like “errors” or “inaccuracies” instead of “hallucinations.” “Hallucination” sounds smart, like we’re AI insiders and know the lingo, but the term is often defined narrowly as a fabrication, which is just one type of error. Customers will be as concerned, if not more concerned, about non-fabricated statements from non-fabricated cases that, despite being real, are still incorrect for the question. “Errors” or “inaccuracies” are much better and more encompassing ways to describe the full range of problems we care about.
Next, let’s consider types of errors and risk of harm from each. Error rates are often just reported as a percentage, which is a binary view – either an answer has an error or it does not, but that’s overly simplistic. It conflates the big differences in risk of harm from different types of errors and ignores the potential benefit of lengthy and nuanced answers that contain a minor error.
There are dozens of ways to categorize errors in LLM-generated answers, but we’ve found three to be most helpful:
- Incorrect references in otherwise correct answers
- Incorrect statements in otherwise correct answers
- Answers that are entirely incorrect
A fourth category of error that sometimes comes up in discussions with customers is about inconsistency, where the system provides a correct answer one time, then later, when the same exact question is submitted, the answer is different and sometimes less complete or incorrect. Minor differences in wording are very common when submitting the same question. Substantial differences are uncommon, but when they do result in an error, the error simply falls into one of the three categories above.
Incorrect references refer to situations where an answer is correct, but the footnote references provided for a statement of law does not stand for the precise proposition of the statement. Fortunately, risk of harm with these types of errors appears to be low, since they’re easy to detect when researchers review the primary law cited. Answers with these types of errors still offer substantial benefit to researchers because they get them to the right answer quickly, often with a lot of nuance about the issues, but the researcher still has to use additional searches or other research techniques to find the best source material.
Incorrect statements in otherwise correct answers are often obvious in the answer. An answer might say the law is X in paragraphs 1 – 4 and then, inexplicably, declare the law is Y in paragraph 5, then go back to stating the law is X in paragraph 6. Risk of harm with these errors also appears to be low, since the inconsistency is obvious and prompts the researcher to dig into the primary law to figure it out. Answers with these types of errors still offer some benefit, since they point the user to highly relevant primary law, explain the issues, and help the researcher with what to look for when reviewing primary law.
Answers that are entirely wrong are more problematic. These are quite rare in our testing, but they do occur. Often a simple check of the primary sources cited will resolve the error quickly, but sometimes additional research is needed beyond that. These answers still offer some benefit to researchers, since they often point to relevant primary law in a way that is more effective and useful than traditional searching, but they also come with greater risk of harm, since the incorrectness of the answer is not obvious, and simply reviewing cited sources does not always resolve the issue.
These sound scary, but researchers have been dealing with this type of issue for ages. For instance, secondary sources can be incredibly helpful for summarizing complex areas of law and offering insights, but they sometimes fail to discuss important nuance, and sometimes the law has changed since they were written. If researchers relied on them alone, without doing further research, they would be at risk of harm, even if they consulted cited primary sources.
Yet we would never tell researchers to avoid using secondary sources because they can sometimes be beautifully written, very convincing, and utterly wrong. What we tell researchers is they can be enormously helpful for research but must be used as part of a sound research process where primary law is reviewed, and tools like KeyCite, Key Numbers, and statutes annotations are used to make sure the researcher has a complete understanding of the law.
Individual research tools have rarely been perfect. Their value has been in improving sound research practices. Stephen Embry captured this idea well in his recent blog post, Gen AI and Law: Perfection Is Not the Point:
“The point is not whether Gen AI can provide perfect answers. It’s whether, given the speed and efficiency of using the tools and their error rates compared to those of humans, we can develop mitigation strategies that reduce errors. That’s what we do with humans. (I.E. read the cases before you cite them, please).”
But if you must check primary resources and engage in sound research practices when using a research tool, is there really any benefit to using it? If it improves overall research times or helps surface important nuance that might otherwise be missed, the answer is yes.
Prior to launching AI-Assisted Research, we knew large language models would not produce answers free of errors 100% of the time, so we asked attorneys if the tool would be valuable even with an occasional error, and if we should we release it now or wait until it was perfect?
Most of the attorneys said, “I want this now.” They saw clear benefits and thought an occasional error was worth it for the extraordinary benefits of the new tool, since they would easily uncover an error when reading through primary law. They said that if they knew the answers were generated by AI, they would never trust them and would verify by checking primary sources. If there was an error, those primary sources (and further standard research checks, like looking at KeyCite flags, statute annotations, etc.) would reveal it. That’s why we put AI in the name of this CoCounsel skill, so researchers would be encouraged to check primary sources.
Our customers have submitted over 1.5 million questions to AI-Assisted Research in Westlaw Precision. Generally, three big research benefits come up in discussions:
- It gives them a helpful overview before diving into primary sources.
- It uncovers sub-issues, related issues, or other nuances they might not have found as quickly with traditional approaches.
- It points them to the best primary sources for the question more quickly and efficiently than traditional methods of research.
Customers have described these benefits with great enthusiasm, telling us AI-Assisted Research “saves hours” and is a “game changer.”
Lawyers know they need to rely on the law when writing a brief or advising a client, and the law lies in primary law documents (cases, statutes, regulations, etc.). Researchers have always known that when they’re looking at something that is not a primary law document, such as a treatise section, a bar journal article, or an answer from AI, they must check the primary law before relying on it to advise a client or write a brief. That’s why we cite to primary law in the answers and why we provide an even greater selection of relevant primary and secondary sources under the answers – to make this checking easy.
But what about the lawyer in the Mata v. Avianca case who used ChatGPT and cited a bunch of non-existent cases? That lawyer submitted his brief without ever reading any of the cases he was citing.
That can’t be the standard for considering the value of products like Westlaw that provide a rich set of research tools that make it easy to check primary sources, understand their validity, and find related material. If the standard were, a user might not read any of the primary law, many high-value research capabilities today would be deemed useless.
The way to dramatically reduce the risk of harm from LLM-based results or any other individual research tool, like secondary sources, is what it has always been: sound research practices.
Jean O’Grady conveyed this beautifully in her recent article on Legaltech Hub:
“Does generative AI pose truly unique risks for legal research? In my opinion, there is no risk that could not be completely mitigated by the use of traditional legal research skills. The only real risk is lawyers losing the ability to read, comprehend and synthesize information from primary sources.”
At Thomson Reuters, we’re continuing to work on ways to reduce all types of errors in generative AI results, and we expect rapid improvement in the coming months. Because of the way large language models work, even with retrieval augmented generation, eliminating errors is difficult, and it’s going to be quite some time before answers are completely free of errors. That’s the bad news.
The good news is that harm from these types of errors can be reduced dramatically with common research practices. It’s why we’re not only investing in generative AI projects. We’re also continuing to build out a full suite of research tools that help with the entire research process because that process will continue to be important.
Even when errors get reduced to just 1%, that will still mean that 100% of answers need to be checked, and thorough research practices employed.
We’re currently involved in two consortium efforts to provide benchmarking for generative AI products. When generative AI products for legal research are tested against these benchmarks, I expect we’ll see the following:
- None of the products will produce answers that are all entirely free of errors.
- All the products will require sound research practices, including checking primary law documents, to reduce risk of harm.
- When sound research practices are employed, the risk of harm from errors in the answers is small and no different in magnitude from the risks we see with traditional research tools like secondary sources or Boolean search.
Even in the age of generative AI, sound research practices remain important and are here to stay. As Aravind Srinivas, CEO and cofounder of Perplexity, said,
“The journey doesn’t end once you get an answer… the journey begins after you get an answer.”
I think Aravind’s statement applies perfectly to legal research and to the art of crafting legal arguments. Even as our teams strive to reduce errors further, we should keep in mind the benefits of generative AI and weigh them against the new and traditional risks of harm in tools that are less than perfect. When used as part of a thorough research process, these new tools offer tremendous benefits with very little risk of harm.
This is a guest post from Mike Dahn, head of Westlaw Product Management, Thomson Reuters.