Project Gaia, led by the BIS Innovation Hub, has demonstrated the power of AI-enabled tools to extract data across different regions and reporting standards
The Bank for International Settlements (BIS) has concluded the first phase of a project using generative artificial intelligence (GenAI) to capture climate-related information published by 187 financial institutions across the globe. Using the technical input from climate risk experts at central banks and supervisory authorities, Project Gaia — led by the BIS Innovation Hub in collaboration with the Bank of Spain, the Deutsche Bundesbank, and the European Central Bank — has demonstrated the power of AI-enabled tools to extract data across different regions and reporting standards.
Due to the lack of global reporting standards, accessing relevant climate-related indicators takes significant effort. In financial institutions’ corporate reports, climate-related data often are buried among other financial and non-financial information. In many cases, information pertaining to one company is split across multiple reports, with relevant information contained in texts, tables, footnotes, and figures.
Project Gaia opens up the possibility of analyzing climate-related indicators at a scale that was not previously feasible. The head of the Innovation Hub Eurosystem Centre, Raphael Auer, spoke to Thomson Reuters Regulatory Intelligence (TRRI) about the project, its findings, and its potential future use in the financial sector.
The following is an edited version of the conversation:
TRRI: From reading the report on the Gaia proof of concept, what you’re doing is capturing information that’s been published in the public domain by banks, either through annual reports or special reports on what they’re doing in terms of climate or net-zero goals — everything that’s in the public domain. Is that a correct understanding?
Raphael Auer: The context of Gaia is that the financial sector as a whole will face climate-related risks. Those could range from a change in the frequency or severity of harsh weather events or other physical risks, as they’re called, or it could result from simple valuation effects. As the economy shifts its structure, there will be associated changes and firm valuations might be somewhat affected.
But from a supervisory perspective in which you have a systemic view, you want to know on balance who’s going to be affected in the first round. Could there be systemic risks? Therefore, central banks, supervisory authorities, and I also think that the financial institutions themselves need accessible high-quality data to model the systemic risk posed by climate change.
There is a lack of global reporting standards. It’s not that there is no data out there, the problem is that there is no publicly available systemic data.
In addition, there is a lack of global reporting standards. It’s not that there is no data out there, the problem is that there is no publicly available systemic data. And very much to the point of your question and as context for the project, we are not creating new data — what we’re doing is capturing all the data that banks or other financial institutions voluntarily publish. And we are then structuring that data.
TRRI: You mentioned a “supervisory perspective”. As you know, in the United States, there’s been a lot of discussion, such as by the Federal Reserve, about its supervision of climate risk at US banks and how much the Fed may want to ask from them. Sometimes, it can be a little controversial. But just in general terms, this tool that you’ve developed, to the extent that it would be used by an individual central bank in its supervisory role, would seem very helpful in that the central bank would be able to collect what’s in the public domain about the banks that it supervises.
Auer: This is a one-time prototype. And so, no central bank has access to it at the moment. But I think there are two aspects: one, is that banks are actually voluntarily disclosing this information, and it’s just not in a standard format; the other, is [the question of] when will a standard emerge in the years ahead. There is a time gap, however, and this may take a few years.
In the meantime, for people that want to do systemic analysis — those could be researchers or people who really want to do some structural analysis — they can do so now instead of waiting five years for the first annual year of data to arrive. One would already have a good set of data with which to work to model the financial stability implications.
TRRI: Since you’ve published the report, have you gotten interest from individual central banks to perhaps use the prototype, use the software in their own supervisory process?
Auer: I wouldn’t know if it would be used in specific processes, but there’s definitely quite some interest in what we’re doing. And not only in the specific context of climate change risk analysis, but also in terms of the general use of generative artificial intelligence (GenAI) in preparing information. Going from unstructured information to structured information — I think that’s a big part of the contribution.
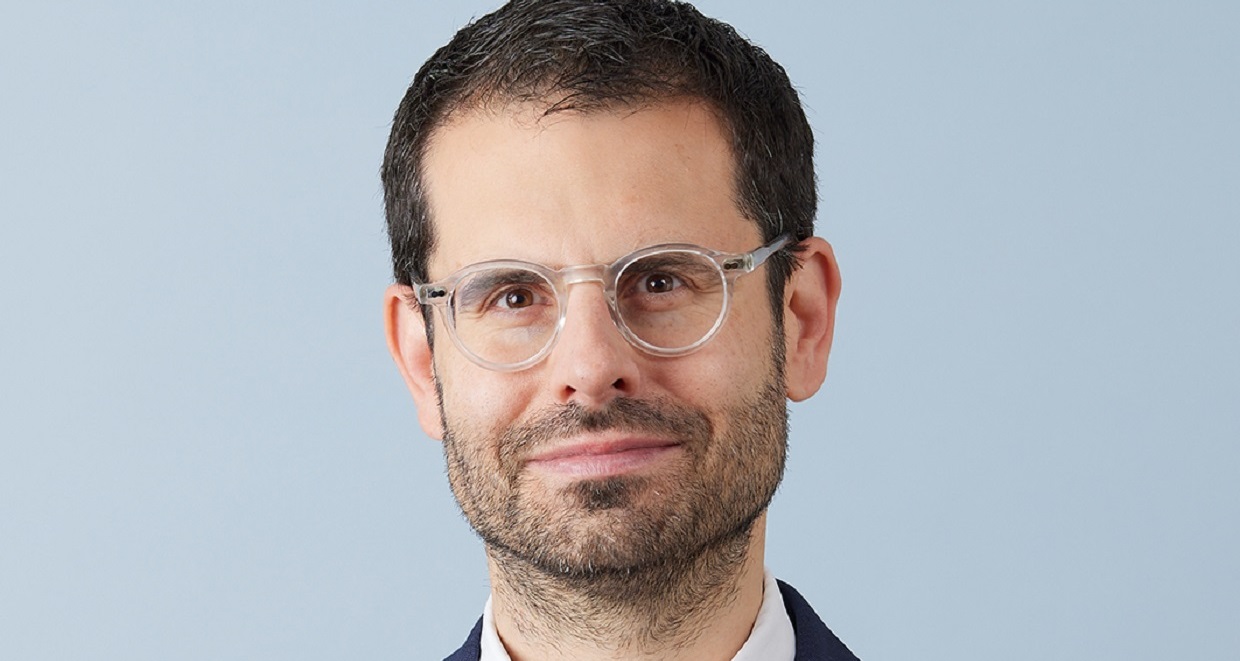
For example, if you think about cyber-risk assessments, that information is always unstructured. Whenever you want to do some type of analysis on this, you need to input it from text into numbers. So, these tools can come in handy. Again, it’s a prototype, and central banks could have an interest in this from a variety of perspectives, but what we see is that there are a lot of people who are asking us to present it.
TRRI: Further along in the report, you talked about looking ahead and possible next steps, and you mentioned that there is the possibility of making the solution publicly available as an open web-based service. Speak to us a little bit about that. How do you see that developing? Who could use it?
Auer: Keep in mind the BIS Innovation Hub spearheads the use of innovative technologies by the central banking community. Therefore, we have a bit of an exploratory and prototyping mandate. What we set out to explore and want to really understand is how the technology works, what would be the use cases that are economically useful, and then explaining that to central banks. Gaia has a very specific objective of how you could use AI via good prompting and other design choices. That’s our first deliverable.
We do not have an operational mandate, but we could develop elements of something that others could then build on for operational use. It’s clear that the BIS would not operate a tool, but given the usefulness of what we did, and given the interest of central banks, I’m optimistic that parts of Gaia — something modelled on an architecture such as this — can be part of the toolkit of climate financial analysis.
TRRI: It seems that when reading your report and those from the private sector, it doesn’t matter whether you’re a regulatory authority or a central bank, or a big commercial bank with millions of clients across the globe, in this area you’re all struggling with the same issues in terms of collecting information that can be comparable. The challenges are the same for both the public and private sectors.
Auer: Yes, I think the interests of the private sector and the public sector are quite aligned when it comes exactly to what we’re doing — and that’s also why we’re doing exactly this. Everybody wants to know the systemic implications, and for that, you need a comprehensive view and a comprehensive data set. There are data gaps, and this is one step towards solving them.
TRRI: And this is where the technology that we have today, in which AI can really help fill in those gaps and problems in collecting that information.
Raphael Auer: However, it needs to be done right. As the report shows, there are hallucinations you need to tackle, and there are wrong values the machine can give you. It’s about learning how to talk to the machine and asking the same thing over and over again, but in different ways. Asking the large language model to literally question itself.
For the full version of this conversation, visit Thomson Reuters Regulatory Intelligence.