Regulators of AI should not make the same mistake as past due process rulings, said a Stanford Law professor at the CodeX FutureLaw conference — AI is not a binary to be applied in the same way in every situation
STANFORD, Calif. — With the rise in generative artificial intelligence (GenAI) usage over the past year, there has been an accompanying interest in regulating the technology. Already, the European Union’s key legislation, the EU AI Act, has instituted some preliminary minimum standards around AI transparency and data privacy.
In the United States, meanwhile, the White House has issued an executive order around safe, secure, and trustworthy AI use and development. And in the legal industry, bar associations in New York, California, Florida, and elsewhere have issued opinions for how AI and GenAI technology should be used by lawyers and legal professionals.
However, as guidance around AI continues to develop, there is one crucial piece to remember, warns Stanford Law School professor Daniel Ho: Binaries are tough to put into policy. AI, and particularly GenAI, isn’t going to be a use-it-or-don’t proposition. As he explained in the opening keynote at the 2024 Stanford CodeX FutureLaw conference, applying AI in the real world necessitates trade-offs that make even well-meaning guidance more complex than it seems.
Lessons from Goldberg
Ho structured his keynote around what he called two provocations. The first is that he worries that some rights-based calls for AI regulation may be falling into the same trap that plagued past rulings around due process.
In his keynote, Ho explained that the 1970 U.S. Supreme Court decision in Goldberg v. Kelly — a ruling that was initially described as “reason in all its splendor” for establishing due process rights — but soon showed the Court that not all cases can be treated the same. “It became incredibly difficult to treat that as a binary on/off switch,” Ho noted.
“We can have policy proposals, but in practice, any deployment instance is going to trade-off these things at the frontier. The question is how we make that transparent… that really requires active science at the frontier of the technology.”
Just six years later, in Mathews v. Eldridge, the Supreme Court needed to scale back that earlier ruling, creating a set of what have been deemed “factors in search of a value” to determine the extent of due process. “It really was the Supreme Court’s lesson in providing a binary rights framework,” Ho said. “We are at risk of making the same mistake when it comes to AI regulation.”
To show one way that mistakes could occur, Ho pointed to a March memo from the U.S. Office of Management and Budget around government agency use of AI. The memo states that “to better address risks from the use of AI, and particularly risks to the rights and safety of the public, all agencies are required to implement minimum practices… to manage risks from safety-impacting AI and rights-impacting AI.”
There are some problems with minimums, however, and those tend to fall into the same traps that the US found in Goldberg, he added. First, government services are vast and varied — the National Parks Service, the Postal Service, and the National Science Foundation all would approach AI in very different ways. And second, AI itself can be integrated in a wide range of ways, scaling from basic process automation to auto-adjudication. Determining one clear line that counts as proper AI for all use cases could be nearly impossible. “That’s the same struggle that Mathews v. Eldridge wrestled with, trying to draw that line,” Ho explained.
In the 1950s, for example, the U.S. Postal Service introduced new technology to help identify zip codes, creating more consistency and efficiency in sorting through the mail. Hypothetically, citizens could claim privacy rights over a machine scanning their mail, but in doing so, especially given the large amounts of mail going through the system, the costs to hire additional humans to sort the mail instead would be astronomical.
Determining trade-offs
And therein lies the second of Ho’s provocations: Moving from principles to practice requires confronting trade-offs. “We can have policy proposals, but in practice, any deployment instance is going to trade-off these things at the frontier,” he explained. “The question is how we make that transparent… that really requires active science at the frontier of the technology.”
Ho described some work Stanford’s Regulation, Evaluation, and Governance Lab (RegLab) performed for the U.S. Department of Labor (while also explaining that he was not speaking for the Department of Labor in his talk). There, RegLab built out a model for AI assistance on document ranking, helping claims examiners determine what to look at first, and span identification, explaining what to look at specifically within a document.
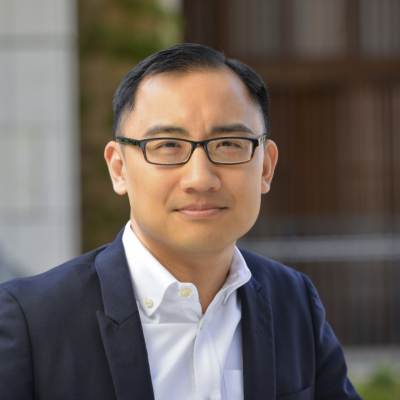
Soon, however, researchers ran into a problem. “The reality of integrating a model like this into a complex bureaucracy means it’s a tiny sliver of what the model actually has to interact with,” Ho explained. Often, engineers may spend a lot of time thinking about the technical details, when they really need to think about the system as a whole, which includes a wider array of people and process factors.
For instance, the document-ranking system necessitated high-fidelity data in order to properly rank the system. However, upping the data quality would require taking examiners off of their current jobs and essentially re-adjudicating the claims in order to assign labels. Or similarly, engineers could make a determination about how much to highlight for examiners within a document, but actually fine-tuning the system would require examiners themselves to explain what is worth or not worth highlighting.
“You really have to think about the trade-off of the resources you have available to create high-fidelity data,” Ho said.
The RegLab team was able to solve these problems through various methods, such as constant testing of how much to highlight as examiners were working through documents. In doing so, they demonstrated a few conclusions about trade-offs in instituting AI in the field, he noted.
First, it is important to have notice & comment periods for anything that is being instituted, Ho said, but “an open question in the field is, how do you do that in a way that is sustainable, cost-effective, and gets input” in an effective way? He pointed to the U.S. Federal Communication Commission’s comment period on net neutrality, which received millions of comments (particularly following a Last Week Tonight with John Oliver TV segment), making it next to impossible to distill what was the general public was saying. Still, there are some potential processes that could leverage GenAI that are “very promising” in this regard, Ho added.
Second, there will be accuracy trade-offs in AI as well, mostly because it’s tough to get an resource-strapped agency to use highly skilled staff to label and audit, Ho explained. While the early EU AI Act said AI should contain no errors, for instance, “that’s going to be extremely costly” to get to that point. At the same time, solely using historical training data, while less costly, could also be problematic as many data sets are riddled with errors themselves, he said.
Third, there are evaluation trade-offs, particularly within the marginal impact of AI assistance, he noted. In some cases, such as the Department of Labor’s document-ranking system, AI will truly be able to help. In other cases, AI’s effect may be more marginal to the point of not being worth it. In the end, however, actually evaluating AI’s effect will take time and effort itself, which also potentially limits its impact.
“It’s really difficult to get away from where the Supreme Court ended up in Mathews v. Eldridge,” Ho said, adding that applying AI isn’t going to be the same in every scenario, and recognizing that fact up-front will give regulators, developers, and users alike an advantage in determining the critical next steps. “In practice, the responsible and really difficult thing we have to do is confront these trade-offs.”